Seven saturdays with singular learning theory
July 2023–
I got a grant to work on introductory resources for singular learning theory (SLT) for six weeks. I’ll spend a little time every Saturday and write about my progress, goals, and lessons learned. (There will be seven Saturdays if I include the Saturdays before the first week and after the last week.)
Contents:
- Prologue: Lining up the project: time and money.
- Saturday 0 (July 22nd): Conceiving the literature review.
- Saturday 1 (July 29th): Or not! Travelling for ICML 2023, project start delayed.
- Saturday 1 (August 5th): Slowly getting back into the swing, project start delayed again.
- Saturday 1 (August 12th): Third time’s the charm! Literature review outline, understanding transformers.
- Saturday 2 (August 19th): Understanding in-context learning and the task diversity paper.
- Saturday 3 (August 26th): Half way!
- Saturday 4 (September 2nd): A brief update, re-evaluating project targets.
- Saturday 5 (September 9th): TODO.
- Saturday 6 (September 15th): TODO.
- Epilogue: TODO (reflecting on the project).
Prologue
In late June, I attended the inaugural SLT and Alignment Summit in Berkeley, California. High on motivation towards the end of the summit, I was desperately looking for some funding so that I could spend some time contributing to research on SLT.
I was lining up a visit to Krueger’s lab for a few months later this year. The start date was initially planned for early August, but it was looking like it would be pushed back to more like September. I could wrap up my RA position at Melbourne by the end of July, and so this left an appealing window of time to work on SLT.
All I needed was funding, and if I was going to catch this opportunity, I needed funding fast. Luckily, two new fast-turnaround funding programs, Lightspeed grants and manifund had just launched and were taking applications. I sketched out my application on the flight home and finished it a few days later, just before the lightspeed deadline. I also sent it to a manifund regrantor (and previous collaborator of mine), Adam Gleave, who liked the project enough to fund it!
So it all lined up perfectly! And I’ll see you on the Saturday before the project!
Saturday 0
Saturday, July 22nd, 2023
I just finished my second last week in my RA role at Melbourne. It’s effectively my final week, because next week I am off to ICML to present my CHAI internship paper. So I spent the week nicely wrapping up my contributions to the project (refactoring and documenting code, documenting next steps, etc.). Satisfying work!
One of the days I was on campus, I met with Edmund and we mapped out some of the key ideas to cover in an SLT literature review. This is the main project I’m going to be working on for the 6 weeks.
There’s not a lot more to say yet, other than perhaps to share this Venn diagram, reproduced from the whiteboard in MathSpace where we sketched out the review:
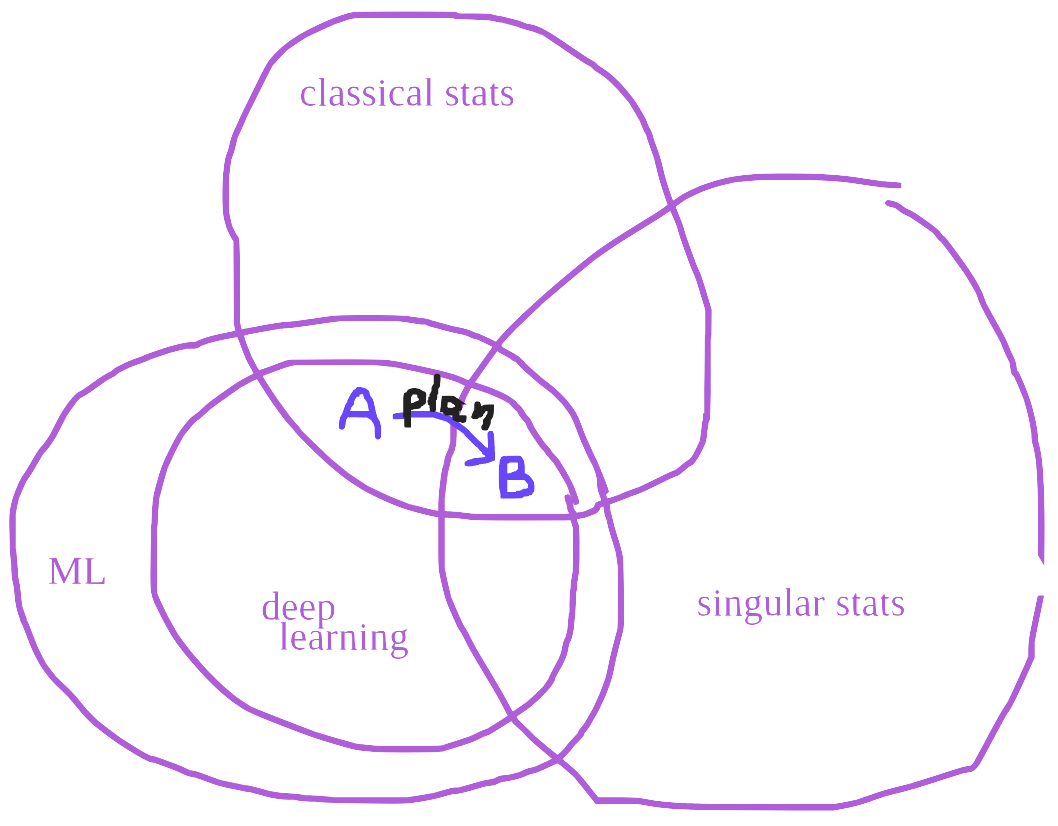
More next week!
Saturday 1 (take 1…)
Saturday, July 29th, 2023
Writing from the AirBnB in Waikiki. I’m not sure how anyone manages to get any work done during conferences. Well, I guess I did get moments here and there, but I spent them working towards a seemingly more urgent deadline. Anyway, I will adjust my actions and my expectations in the future (like a good Fristonian). For now, I will extend the grant by a week. (I’ll figure out what to do about the clash with Cambridge later).
I can share a lesson that I started to learn at the SLT summit and that has been reinforced here at ICML: research is not a personal enterprise. Publishing a paper at a conference isn’t just a matter of dumping facts into some abstract research record with your name attached to them. The positive accumulation of human knowledge that Sarton told me about is actually implemented as, wouldn’t you know it, a community of humans. The conversations and connections at the conference are as important (perhaps more important) than the posters and the oral presentations.
This lesson is more general than about the SLT project, but it has implications. If we’re going to get SLT off the ground as a research program, we need to get SLT into the hearts and minds of the researchers, not (just) into NeurIPS, ICML, and ICLR.
One other update on the SLT project. Dan invited me to work with himself, Susan, Jesse, and Liam on an experimental project attempting to explain the phase transition to in-context learning in transformers using SLT.
Goals for next week:
- Get home safe.
- Get to work!
Saturday 1 (take 2…)
Saturday, August 5th, 2023
I made a slow start this week. The first part of the week was spent travelling home, and the second catching up on some admin. I will probably not count this week either, and try to start again next week! Either way, now the project is finally in motion!
On the SLT literature review:
- I sketched out most of a literature review. To discuss with Edmund next week.
- We found a recent survey of SLT by Watanabe himself, which is clearly closely related work. We will have to plan our audience and scope carefully to make sure we are contributing something novel here.
- I made plans for a regular in-person meeting with Edmund to discuss the project.
- By the first such meeting (Tuesday), I want to have a fleshed out sketch and reading list to discuss with Edmund. Next week I need to start reading and writing like mad!
On the in-context learning project:
- I studied the main paper on in-context learning emerging at a task diversity threshold. I presented it to the group.
- I had to brush up on some transformer basics. I haven’t really paid deep attention to the architecture before, that will have to change for this project.
- We planned to meet again in two weeks. By then, I want to have looked at more of the relevant papers and I want to have a clear plan for what experiments we could run.
(On side projects:)
- I finished, submitted, and published my responsibility letter.
- The NeurIPS reviews came in (for the two papers I submitted based on my thesis). My reviews are remarkably mixed, with ratings ranging from 3/10 to 10/10(!). I’m marginally above the acceptance threshold on average, but it’s probably not enough. Luckily, I have a chance to respond to reviewers over the next week and I am optimistic that I might be able to sway some of the low ones upwards.
Saturday 1 (third time’s the charm!)
Saturday, August 12th, 2023
OK, I decided to make this past week the official first week of the 6-week project. That’s final. This worked out for the best because:
- By committing to work another week later, I feel much better about not having gotten much time to work on the project last week. I’m giving myself a second chance.
- When I finally submitted my UK visa application (for the Cambridge visit in September), they told me it should take 3 to 6 weeks! I was previously expecting a 3 week turnaround based on my (optimistic?) reading of the UK immigration website. I guess that might still happen, but this gives me a second reason to delay the start/end of the 6 week project (because I can plan to start in Cambridge a couple of weeks later anyway.)
Anyway, since I was able to spend most of my attention on research this week, things really got into a swing!
For the SLT literature review:
- The main milestone I achieved this week was refining the sketch from last week with Edmund and Ben (another PhD student considering joining the project) and then turning this sketch into most of a more detailed outline of the whole review.
- I also dug up my reading list from my Master’s project. The Master’s list had nearly 1500 papers on it. I filtered this down to ~630 readings of potential relevance to this review (as opposed to the topics I actually wrote my thesis on), ~350 of which are potentially directly relevant to singular learning theory (the rest are potentially relevant to the classical statistics background or other background topics). I’m pretty proud of the SLT list but it’s not exhaustive, and I hope we can soon make it more complete through (1) integrating Edmund’s lists, (2) searching for recent papers and on specific topics, and (3) combing through references to see if we can find any important contributions I missed.
- I reflected somewhat on the goals of the literature review. There
are two or three audiences that are coalescing in my mind:
- Existing members of the SLTxAlignment community. These are the people who attended the Summit, or learned about SLT from the Alignment Forum or from an alignment podcast or something. They are probably pretty familiar with deep learning, but they may or may not have a background in classical statistics or in mathematics. For these people, we should not assume such backgrounds either technically or even in the paradigm we write under—the implicit ways of thinking in the fields of mathematics and statistics need to be made somewhat explicit?). We should also be sure to make clear the connection (or lack thereof) between the research program we are sketching and the alignment problem.
- Deep learning people, who care about theory that can give them leverage on understanding deep learning, but, like the previous group, they are not necessarily already familiar with the paradigms of classical statistics. Likewise for these people we need to make explicit the connections between SLT and what they want (leverage on understanding learning) in our framing and discussion of various results.
- Deep learning people with a background in statistics (surely such people exist). I guess catering to the previous two groups is going to cover this one?
- Next week: I’m looking forward to discussing the outline and the reading list with Ben and Edmund next week. I’ll get their input on both (including, hopefully, integrating their own reading lists into my own). Then, I guess it will be time to get writing and citing!
For the in-context learning project:
- The main milestone from the last week is that I would say I now have
a strong understanding of the transformer architecture. I found several
useful resources to get to this point:
- Several brief YouTube lectures that covered the basic elements (encoder, decoder, attention, positional encoding) at a high/intuitive level, without details. At this point I felt like I knew what the elements where but not in detail and I didn’t know how they fit together.
- A recent StatQuest video on transformers. If you look past the childish format, this was actually really informative and impressive (I think this channel’s videos are generally really great for explaining ML topics clearly). Anyway at this point I felt like I knew a bit more about how the elements fit together, but I needed to go through it once or twice more, slowly, in detail, for it to really stick.
- Andrej Karpathy’s Stanford lecture on transformers. This contained interesting historical notes that helped me understand the research context of transformers.
- Andrej Karpathy’s pytorch tutorial on building a tiny character-based decode-only transfomer. I followed this tutorial in detail, step by step, and afterwards I felt I had a pretty strong understanding of the architecture.
- Phuong and Hutter, 2022, “Formal algorithms for transformers” (arXiv). After following the Karpathy tutorial, this was transparent to me, which told me I had a good understanding of the architecture now.
- For next week, we’re still aiming to have a better understanding of
the in-context learning literature and to have an idea of what
experiments to run by our meeting (probably Friday). I think I’ll
prioritise two things:
- Reading the task diversity paper again and attempting to set up a pico-scale replication I can run on my M2 macbook air, using the Karpathy tutorial code as a starting point.
- Forming a broad understanding of the in-context learning literature, like by starting a reading list and reading the foundational papers (the GPT-2 paper?) and finding + watching some lectures/talks on the topic.
This week I also experimented with working from various locations on different days.
- I spent 2 days working from home. I’ve never been a big fan of working from home. It’s nice to have a stable work environment (including all of my textbooks and notebooks). However, I have struggled with separating work time and other time when working primarily from home—in particular, I struggle to get started in the morning and when I do get on a roll I struggle to step away from work in the evening. It’s something I’m working on, but in the mean time, I figure I can also look for ways to get out of the house for the workday.
- I spent 2 days working at the Melbourne campus. Since I no longer have a desk (since I wrapped up my RA position at Melbourne) I mainly wandered around to random study spots and worked on my laptop. I also took the opportunity to line up meetings with Edmund and Ben to discuss the SLT literature review in person. I also ran into Dan on campus! This was surreal because for the two years while he was my Master’s supervisor, I interacted with him almost entirely virtually. Sigh—it’s nice to have a place to go to work.
- I spent 1 day at the Deakin campus near where I live—I was there to meet a local AI Safety researcher and co-signatory of the Australians for AI Safety letter, Prof. Richard Dazeley. I wanted to meet Dazeley to learn about his research and his thoughts on existential risk from AI. We had lunch and he also showed me around the campus, which is very open and natural, and I can see myself spending some time there in the future (both as a nice place away from home to visit/work and as a potential place to apply for faculty jobs in the more distant future).
On the topic of productivity, last week I recruited a few colleagues and a few of my peers from Master’s to join me for twice-weekly virtual ‘shut up and write’ sessions (wherein we join a voice call, but are mostly silently writing/working, using the pomodoro technique with a screen-shared virtual timer). I ran two such sessions during the week. Ben and Edmund also joined my end of the call in-person for (part of) both sessions.
The sessions were pretty effective! This is not such a surprise, since I was simply reviving a practice I had started with some of these peers during my own Master’s degree. At one point, we were doing these sessions once or twice daily, and I credit them with a substantial portion of the words in my thesis (not to mention the many more words ultimately cut from earlier drafts of my thesis). I think this is a pretty powerful system I have here, and I should try to find a way to keep it as part of my work life going forward.
(On side projects:)
- I spent some of my train commutes reading and reflecting upon my NeurIPS reviews, and I drafted and posted the rebuttals themselves on Thursday. As I said last week, for both papers, there was a wide range of reviewer ratings. Now that I have read the reviews, it seems that most of the reviewers actually mostly agree on where the strengths/weaknesses of the paper are, they just disagree on whether the contribution is sufficient for acceptance. The main concern is along the lines I was expecting—due to limited time in my thesis project, I studied a simple (single-hidden-layer) network architecture. It seems reviewers mainly disagree on whether or not this is enough. In my rebuttals I have tried to point out that the contributions are not only specialised to this architecture but also form part of the picture for more sophisticated architectures with layers as subcomponents. We’ll see where the ACs think the line falls.
- I lined up to present a talk on Singular Learning Theory, Developmental Interpretability, and AI Safety research at Melbourne. The talk is next week at the monthly meet-up for AI Safety Melbourne. This should be a good chance to meet local people interested in AI Safety, or at least to introduce myself to them. I’m looking forward to it! I’ll report back next week.
Saturday 2
Saturday, August 19th, 2023
This week, as Edmund worked towards the AAAI submission deadline for another paper, I mainly focussed on the in-context learning project:
I made a start in the in-context learning literature:
- I read Dong et
al., 2023, “A survey on in-context learning”. This turned out
to be mainly focussed on applications of in-context learning with a
large language model as opposed to a specialised supervised learning
set-up. This led me to realise a distinction:
- There is in-context learning as a learning paradigm: the literature survey spends most of its efforts breaking down the design choices involved in preparing a foundation model for effective in-context learning and prompting it in the optimal way to elicit competitive learning performance for a learning problem, where what yo care about is performance on that learning problem.
- Then there is in-context learning as an emergent phenomenon: There was a comparatively small discussion of in-context learning itself as an interesting capability of foundation models that emerges from unsupervised language modelling. However, there were some leads into studies of variables affecting its emergence (such as amount and kind of training data) and several studies attempting to explain the mechanism behind the capability.
- I started a reading list with the interesting papers identified from the literature survey. In total, there are about 50 sources in the reading list now, though that includes some of the lectures, tutorials, and blog posts I found useful for understanding transformers previously. In addition to the papers I identified based on the literature survey, Dan had also sent through a short list (of about 6 papers) relevant to the project. I had identified about 5 of these 6, which to me indicates that the literature survey was pretty broad but not comprehensive search. I should continue to explore for a bit longer to make sure I don’t miss anything. Of course, part of the reason the literature survey may have missed some of these papers is that they are very recent. As this is a very active area of research I should make sure to keep abreast of new papers dropping on the arXiv.
- I read Dong et
al., 2023, “A survey on in-context learning”. This turned out
to be mainly focussed on applications of in-context learning with a
large language model as opposed to a specialised supervised learning
set-up. This led me to realise a distinction:
I continued to improve my understanding of transformers, with an emphasis on recent work on their mechanistic interpretability. Highlights include:
- Finding this Stanford lecture by Chris Olah giving a detailed ovreview of the induction heads research (prior to its publication).
- Finding more walkthroughs by Neel Nanda on transformers and various mechanistic interpretability topics (I have only watched two so far but plan to watch more going forward).
A specific insight included understanding the role of the ‘residual stream’ as opposed to viewing the primary pathway through the transformer as through a sequence of attention/compute blocks. Actually, it seems that people think about both of these perspectives as well as everything in between, that is, considering the various possible pathways through some transformer blocks and around others.
I attempted to understand in detail the specific architecture used for the paper we are studying (Raventós et al., 2023). In this paper the authors consider an in-context regression problem, where a transformer is used to predict sequences of the form where and . and are sampled from standard normal distributions. How the ‘task’ is chosen is varied in the paper’s experiments—the details are quite important to the paper and our project but let me defer an explanation to a future post or refer readers to the paper. For now let me just share that I spent a day this week studying the published code understanding exactly how the authors framed this regression problem as an in-context learning problem.
The authors chose to use a decode-only transformer with a sequence of inputs based on interleaving input and output values. I suppose this makes sense because of the emphasis on in-context learning, which happens in practice in decode-only transformers. But it’s an awkward setup for at least the reason that the transformer will also have to predict the inputs, which is not essential to the task (though it shouldn’t take much capacity, since the optimal prediction will be the zero vector for i.i.d. standard Gaussian inputs). Thus framing the problem for an encode-decode architecture (or, more simply yet, a non-sequential prediction task using a feed-forward or even a straight-up perceptron architecture) would defeat the purpose.
How to encode the sequence of inputs and outputs, then? In practice, the input to a decode-only transformer is a sequence of one-hot encoded vocabulary elements (or, for efficiency, just their indices) and in-context learning problems are encoded using some textual boilerplate. In this paper, thankfully! the authors stripped out the language component and just encode the input vectors and output scalars directly as vectors in place of the one-hot token encodings. There is the small matter of dimensionality ( while ) which they handle by embedding both inputs and outputs in a joint space (that is, and ).
What about the transformer outputs? Since tokens are not one-hot encoded, rather than logits trained with cross-entropy loss, the transformer outputs are -vectors using the same encoding format as the input, and these are trained by mean squared error loss. In particular, as far as I can tell, the loss penalises predicted inputs as well as penalising deviations from zero in the tail of the predicted output vectors.
After understanding the paper to this extent, and with my deeper understanding of the transformer architecture, I felt ready to code up a basic architecture and task. I spent most of the rest of the week on this and got to the point where I have a small transformer training against simple synthetic data on my M2 macbook air, using PyTorch with MPS hardware acceleration. The paper quotes 4 TPU hours per training run (e.g. to get one data point in their figures), and by my rough estimates, such an experiment would take around 16 hours on my laptop (not counting inevitable thermal throttling, and with a lot of uncertainty in how the authors got their quote). This is not as bad as I thought! A reasonable starting point, from which we can work on:
- moving to more efficient compute;
- improving the efficiency of the code (e.g. flash attention?); and
- once we have a proof of concept, we might be able to economise on architecture size and so on while preserving the phenomena we are studying.
The next steps on this project are clear:
- Continue to explore the literature on the phenomenon of in-context learning.
- Continue to develop the experimental codebase including more tasks, baseline algorithms, and tools for evaluation, aiming to reproduce the apparent phase transition as soon as possible.
- Continue to scale compute experiments and improve algorithms to make the workflow progressively more efficient.
The SLT literature review was basically on hiatus. However, I did have one meeting with Ben to go through the reading list and identify areas where it needs expansion. Next week we’ll pick this back up with roughly the same goals as last week.
(On side projects:)
- NeurIPS papers: A few of my reviewers have slightly increased their review score. In one case from borderline reject (4) to borderline accept (5), and for the other paper from borderline accept (5) to weak accept (6). Pending any further score changes, I have 4/5 and 3/4 reviewers recommending acceptance (borderline or above) of the papers respectively, with one reviewer recommending rejection in each case. All up, I think I have a pretty good chance to get one or both accepted now (but not a sure thing, still pretty uncertain due to lack of experience here). So, looking forward to finding out late in September.
- The AI Safety Melbourne talk went well, I think. It was a casual discussion about SLT and developmental interpretability research (and their safety applications and implications), and the researchers and research groups behind it. The audience seemed pretty positive about the research ideas. I enjoyed meeting the attendees and chatting with them about AI Safety research in Australia. So, I think this was a success.
- I don’t think I have any pressing side projects over the next week… I should be able to return to some less urgent projects from my limitless TODO list. I will let you know how this goes.
Saturday 3
Saturday, August 26th, 2023
Today marks the project half way point! There is still so much to do. But I’m also positive about the start I’ve made.
On the in-context learning project:
It’s time to tell you a little more about the experiments we are replicating, namely the findings of (Raventós et al., 2023). The authors of this paper train transformers on an in-context linear regression task where, during training, for each context, the true regression solution (vector of coefficients) is drawn uniformly at random from a fixed, finite number of possible regression solutions. The basic finding in this paper is that when transformers are trained on such a data source, their learned in-context learning behaviour undergoes a phase transition as we vary :
- Discrete prior: For small , the transformer appears to behave in-context as a Bayes-optimal learner with a uniform prior over the possible solution vectors. That is, it uses the context primarily to perform a Bayesian calculation about which of the solution vectors is generating the context, and then it makes new predictions based on the posterior predictive distribution.
- Gaussian prior: For large , the transformer appears to behave in-context as a Bayes-optimal learner with a Gaussian prior over all possible solution vectors (i.e., in-context ridge regression). The Gaussian prior matches the way the true solution vectors are sampled in the first place, but, importantly, this means the transformer will include hypothetical solution vectors in-context that it has never been trained to consider.
The authors frame this as ‘the emergence of in-context learning’. It seems to me that in both cases the transformer is learning in-context, and this is just an interesting change in the specific in-context learning algorithm (or prior) it is using. Regardless of framing, we want to carefully study this apparent phase transition as a testbed for some of our theoretical predictions about deep learning and our nascent developmental interpretability ideas, methods, and tooling.
The replication of this phase transition is the current objective. On this front we are making good progress so far:
- I implemented and tested the Bayes-optimal in-context learning algorithms described in the paper (with the discrete and Gaussian priors). These algorithms return what the Bayes-optimal predictions would be, which we can then compare to our transformer’s predictions to test if it appears to be implementing something computationally equivalent to one of these algorithms.
- As discussed previously, the full training runs discussed in the paper use more compute than a laptop can muster in an afternoon. I dusted off my GTX 1050 and ran some 11 hour training runs over my LAN (this is what took 4hrs on a TPU according to the paper, and estimated 16 hours on my M2 air before accounting for thermal throttling).
- A funny story is that, for the first run, I was not getting very
impressive performance, and it turns out that I had accidentally
forgotten to wrap the list of transformer layers inside an
nn.ModuleList
. Consequently thetorch
introspection was not finding these layers as a trainable part of the model… In other words, my GPU spent 11 hours very thoroughly and patiently trying to find embedding and unembedding transforms that would get good in-context learning performance out of a randomly initialised 8-layer transformer. Oops! - For the second run, with this issue cleared up, the transformer surpassed the Gaussian prior baseline and got most of the way to the discrete baseline, but not quite all the way. I was hoping for a clearer result, and hopefully the learning performance can be improved. It is still left to implement a learning rate schedule used by the authors of the task diversity paper, so maybe that will make the difference.
Going forward I’ll be working with Jesse to scale up these experiments—he has already integrated my transformer and baseline code into his more sophisticated training and checkpointing pipeline, so, more results should be avilable soon.
I met with Liam to discuss transformer algorithms, and we briefly looked at two important papers on the topic of in-context linear regression in transformers:
- Akyürek et al., 2022, “What learning algorithm is in-context learning? Investigations with linear models”—a construction of transformer weights for performing in-context least-squares regression via gradient descent or directly solving the normal equations (but using quite deep transformers).
- von Oswald et al., 2022, “Transformers learn in-context by gradient descent”—some shallower and simpler constructions for in-context regression via gradient descent steps, and experiments supporting the claim that these algorithms are actually learned by real transformers.
In the coming weeks I may need to take a deeper dive into these papers and their constructions. We are also looking at potentially extending their work to investigate simpler constructions for in-context explicit ridge regression and for the discrete baseline, though there are also some more papers on my list I should take a look at before we spend a lot of effort there.
I’ve made some more progress through Neel Nanda’s walkthroughs, this week working through part of his walkthrough of the mathematical framework for transformer circuits paper. I’m about 2 hours in now, and so far it’s a good summary and explanation of some of the things I’ve picked up from my broader study of transformers, including a couple of new insights for me, or points I didn’t grasp last time I saw them in passing but Neel Nanda does a good job of explaining them in detail here.
Next steps for this project? Actually, I think I should slow down a little on this project over the next week. I can continue to improve evaluation metrics, look into learning rate schedules, and take a look at more papers, but the project that seems more urgent right now would be the SLT literature review.
On the SLT literature review project:
Early last week Ben and Edmund and I met to discuss the project. It was a useful meeting that clarified for me some distinctions such as between algebraic statistics and singular learning theory, and we found a good graduate-level theoretical statistics reference (Shao, 1999, Mathematical Statistics, or actually there is also a second edition). But I think the most helpful discussion was around communication goals and audience for the project. We still haven’t fully clarified this thinking, but made meaningful progress I think. I will keep working on that and aim to document next week.
I didn’t get around to doing any writing. I did aim to spend Tuesday’s Shut Up and Write writing for this project, but couldn’t make a start, so fell back on writing more code for the ICL project. I should probably commit to spending both writing sessions next week writing (as well as substantially more time than that). I know I just need to get started and once I get over the inertia it will be great for the project.
I did successfully manage to start getting into the weeds—starting with a close technical read through of Edmund’s paper on RLCT estimation (preprint now on arXiv!). I’m not actually up to the estimation part yet because I’ve spent so much time stepping through all of the definitions and background. Much of the discussion here is relevant to the literature review project and it’s getting me motivated to get writing our more accessible version for the literature review!
Next steps: Get writing! Just, write something!
(On side projects:)
NeurIPS papers! I thought everything was settled last week, however, there is an update: the night before the end of the discussion period, my paper 1 reviewer recommending rejection posted a message on OpenReview. They had evidently been speaking with my other reviewers, and had a number of additional questions, mainly about connections to deep learning topics like pruning. I stayed up to around midnight to respond (the discussion period ending at I think 3am here), and the next morning I was pleased to wake up to find that the reviewer had upgraded their score from 3 to 7! So the final scores for paper 1 are 5/5/6/7/10, and it now seems reasonably likely to me that this one will be accepted. (With 4/6/6/7 and low-ish confidences, Paper 2 still seems like a coin toss to me.) I’ll know for sure in a month—can’t wait!
As for other side projects, I spent some time thinking about the possibility of taking on my own research students in the near future. Here’s some of my thinking:
- A general pattern in my life is that after learning something I like to pretty quickly jump into teaching it (e.g. private tutoring after highschool, undergraduate tutoring before graduating). I find this work rewarding and valuable for refining my skills and forging relationships, and I have received positive feedback from multiple students on being an effective and helpful teacher.
- Recently what I have been learning, albeit in a primary experiential way, is how to research. In this process I have also been helped by a number of junior researchers (PhD students). While I’m not yet a PhD student myself, I think I could offer valuable advice and supervision to an undergraduate or master’s student. There are actually a number of project ideas I have come up with during my research so far, but haven’t had the time to dig into, that could potentially be suitable for such students.
- There is the small matter of finding and funding such students. Some
standard options such as the following are a little out of reach:
- becoming a professor (need my PhD);
- joining SERI MATS as a mentor (need more community rep);
- joining a group that takes interns (CHAI, Krueger’s lab; working on it); or
- replicating such a program in Melbourne (maybe soon?).
- lower my expectations on how much time and effort the students will put in to my projects; and
- fully devote myself to supporting the students, leaning more towards collaboration than mere supervision.
- So, my next step on this new side-project of ‘becoming a research mentor’ is to write up short descriptions of these projects to advertise on my website and in local EA/AI Safety channels.
Speaking of my Cambridge visit, I received my visa to work in the UK this week! In the end, it took around 10 business days—even less than the 3 week optimistic estimate, and of course far short of the 6 weeks I feared. A great outcome, that helps me plan my travel, you know, not right before the travel. Anyway, I’m one step closer to Cambridge! Of course, that also means I’m one step closer to the end of this grant—another reminder to keep up the pace!
Saturday 4
Saturday, September 2nd, 2023
Sorry, I don’t have time to write a full detailed update this week. Here’s a brief update.
The literature review project is going okay:
- I’ve started grinding through the long and agonising journey towards actually getting words on the page. I have an introduction and contents page to circulate for feedback on the overall high-level framing of the review.
- It seems difficult to imagine that I’d have a completed draft by the
end of week six, but I think this is not terrible because.
- The main reason I haven’t made as much progress as I would have liked is because I have contributed a bunch of time to the in-context learning project, which has itself been a valid use of my time.
- We can still realistically aim to create, by the end of the period, a less formal and polished resource for ciculation in the SLT research network—capturing a significant fraction of the utility we aimed to achieve with the completed review (in particular, the main time-sensitive part).
- I will continue allocating spare time to this project after week 6, to eventually finish the review and publish it more broadly. So, the work towards this goal will by no means be wasted even if at the end of week 6 there is no completed review yet.
The in-context learning project seems to be progressing smoothly, at least as far as the first milestone—replicating the phase transition—goes. We have conducted a few training runs and appear to be getting similar results to the plot in the main figure in the task diversity paper so far. It remains to conduct a full set of training runs and compare our results. After that, of course, we have to start the SLT-based analysis of the phase transition.
Until next week!
Saturday 5
Saturday, September 9th, 2023
TODO.
Saturday 6
Saturday, September 15th, 2023
TODO.
Epilogue
TODO.